Once again we have some nice results to share! Today we look at Twitter’s ReTweet graph, based on the same data set which was described in Teaser #3. The ReTweet graph was extracted from Jure Leskovec’s Twitter Sample, applying a simple RT @username filter (thereby ignoring “dark retweets”).
The resulting ReTweet graph comprises 826,104 users with 2,286,416 edges. Just considering the ReTweet frequencies (i.e., how often user A retweeted user B), we aggregated some average similarity scores. Firstly, we collected all hash tags for each user separately and represented the user by the resulting hash tag context vector (i.e. each component of a user’s context vector contains the number of tweets in which the user applied the corresponding hash tag). Thus we can calculated the cosine similarity between pairs of users, based on the corresponding context vectors. Averaging these similarity scores per retweet frequency, we obtained the following plot (excluding self retweets):
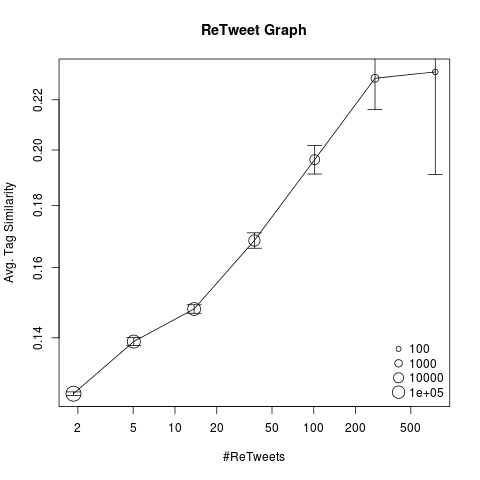
As we can see: Pairs of users who retweet one the other more frequently, tend to be more similar with respect to the corresponding hash tag usage. This is not surprising, but nevertheless, nice to see. Please note that the plots are log-log scaled and retweet freqencies are binned logarithmically.
Secondly, we extracted geo locations for Twitter users and calculated the average geographic distance of user pairs, relative to the corresponding retweet count:
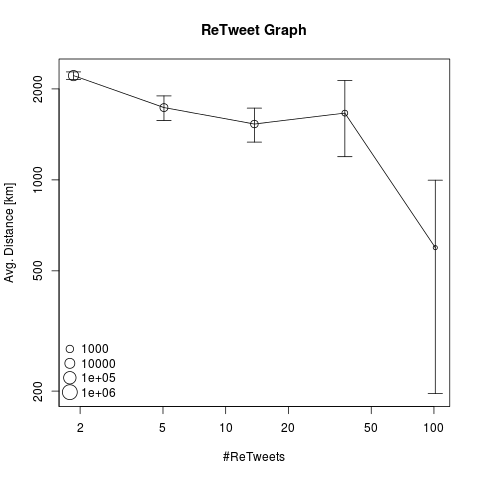
These results are not as clear as in the case of hash tag similarity, but nevertheless, we can observe the tendency of user pairs with higher retweet counts being more closely located. It is worth noting that the global average geographic distance of all users is 7,484 Kilometres and thus already low retweet frequencies yield significantly lower average distances. For your convenience, we also show the linear scale plot:
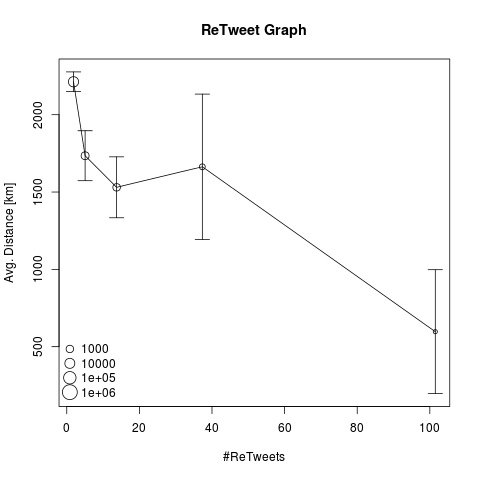
But finally, the interesting part: Again, we heuristically determined given names for Twitter users by matching the user name with our list of known names. We thus collected names for 179,260 users, having 111,204 links in the ReTweet graph (excluding self retweets). We than calculated the average name similarity of user pairs based on the name co-occurrence graph derived from the English Wikipedia corpus (as described in the Nameling papers):
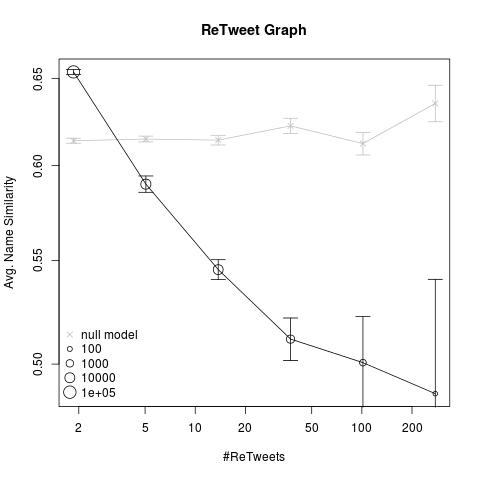
The result is rather unexpected: The average name similarity decreases with increasing retweet counts! That is, spontaneous retweets are more likely among users with similar names and user pairs which retweet often tend to have less similar names.
At this point, further investigation is due. Maybe these results are artefacts induced by the applied name similarity function. But other hypothesis may also support these observations. Higher average name similarity for low retweet counts can be explained by assuming that spontaneous retweets are more likely related to topics which are relevant to the retweeting user’s cultural background (e.g. local events, TV shows, etc.) and for user pairs who retweet often, the name correlated relations are less important, as these users share some focused interest (e.g. Recommender Systems).
These are of course only speculations and we welcome you to discuss these observations either via Twitter or in our forum!
Happy number crunching!